Physical and Intrinsic Security of Embedded Neural Networks
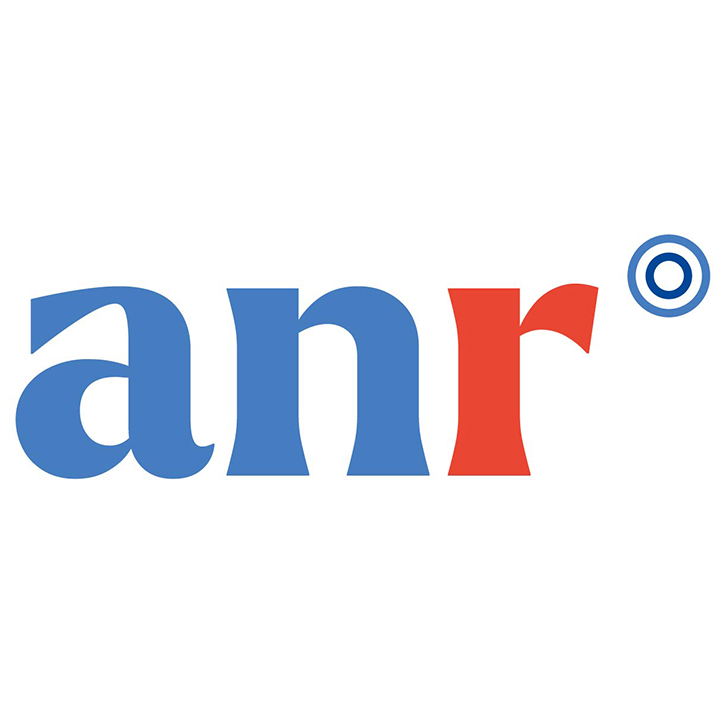
PICTURE is a 42 months French funded project, selected by the ANR (National Agency for Research) through the call AAPG 2020 (ANR-20-CE39-0013). The project started research activities dedicated to the security of embedded artificial intelligence, the 1st February 2021 for 42 months.
The project is coordinated by CEA-LETI (Pierre-Alain MOELLIC) and gathers Mines Saint-Etienne, STMicroelectronics and IDEMIA.

CONTEXT
Machine Learning models are expected to be included in numerous devices, which can be hacked with an extensive overall attack surface, because of critical flaws intrinsically related to the Machine Learning algorithms as much as their implementation in physically accessible devices.
Then, the security of embedded ML models can be seen through the two sides of a same coin. On one side, an impressive body of publications raises algorithmic threats that could have disastrous impacts on the development and durability of ML models by targeting their integrity, confidentiality or accessibility. On the other side, physical attacks (more particularly side-channel – SCA – and fault injection analysis – FIA) for embedded ML models is a relatively new topic with handful of works that have the strong merit to pave the way to extensive experimentation with more realistic and complex frameworks.
As widely admitted by the Machine Learning and Security communities, these two sides are still handled too separately. With PICTURE, our main hypothesis is the exploitation of a joined attack surface, i.e. by combining algorithmic attacks and physical attacks, in order to optimally analyze the upcoming threats targeting critical ML embedded systems as well as design efficient defense schemes.